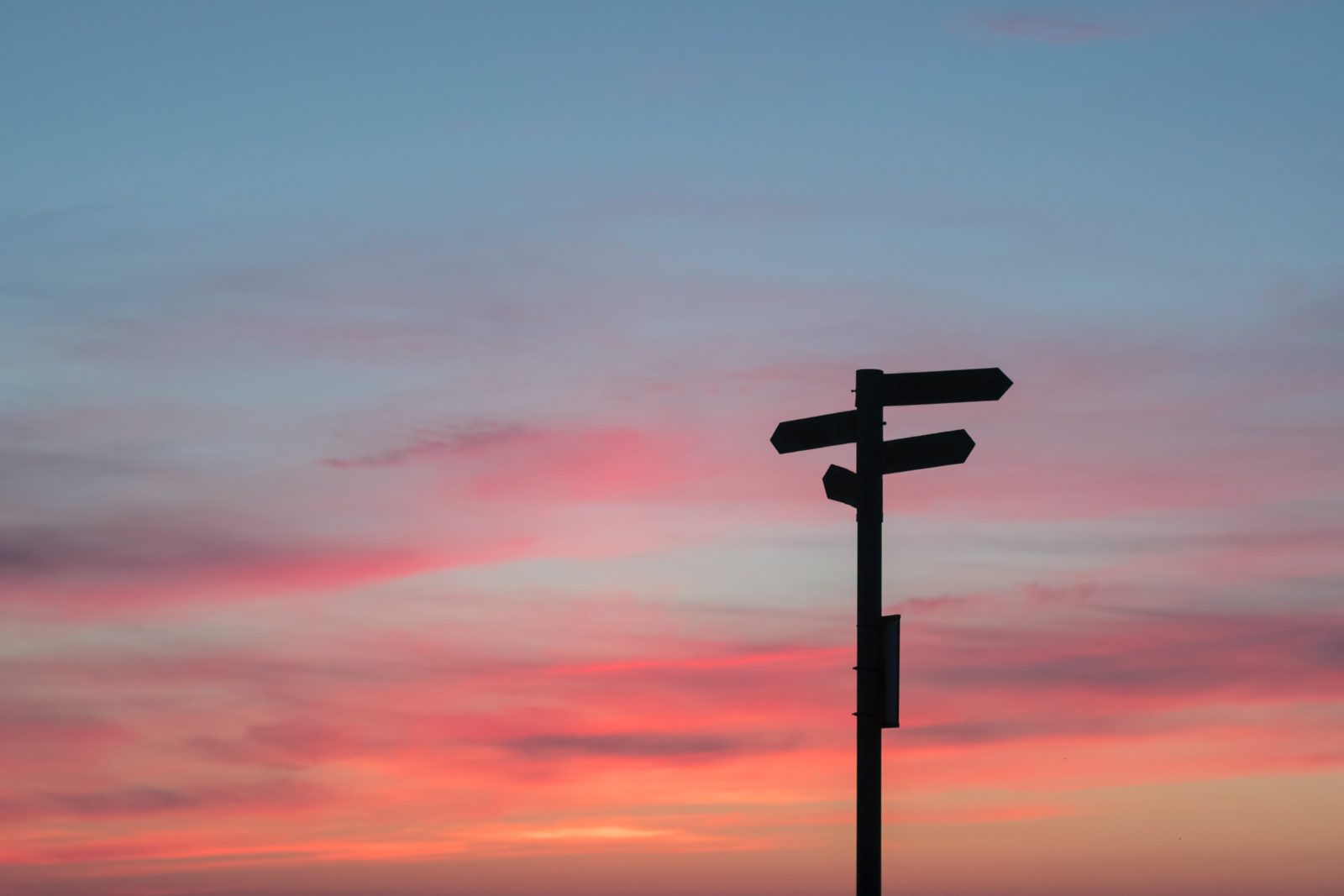
Imagine you’re walking down a corridor at Shackleton & Scott, a FinTech start-up. Either side of you, development teams are hard at work estimating their next sprint. You’ve been told there’s been a huge variation in the accuracy of forecasts between teams lately, which leads to unpredictability, and underwhelming releases to the market. Leadership has asked you to find out why.
The answer lies in the pages of Noise, the latest book from Daniel Kahneman, Nobel Prize-winning author of hugely influential Thinking, Fast & Slow. Along with his co-authors Olivier Sibony and Cass R Sunstein, Kahneman argues that all variability in decision-making is caused by either bias or noise. Whilst bias is relatively well understood as a phenomenon, and active steps are being taken across society to mitigate its effects, comparatively little is known about noise. The book, therefore, poses the following questions: what is noise, why is it important, and how can we improve our decision-making by turning down the volume?
This post will attempt to achieve three things:
1. To highlight the most useful ideas in Noise. The book is nearly 500 pages long, highly repetitive, and full of lengthy examples parochial to US readers (in particular, the machinations of the US Justice and Healthcare systems). There are undoubtedly pearls of wisdom nestled in its pages, but finding them can feel like a slog. This post should help save the reader valuable time.
2. To pose answers to questions raised by the book that fall outside the authors’ argument.
3. To apply the ideas in the book to recognisable, real-world situations.
Lesson One: There Are Different Types of Noise
Let’s start with some definitions. In the book, noise is defined as “undesirable variability in judgments of the same problem”. That’s to say, noise is a symptom of unpredictable error that’s hard to explain away. Noise dilutes accuracy, and promotes disagreement. Indeed, the authors liken noise to an invisible enemy, and point out that “taking it on can therefore only yield an invisible victory”. This is unfortunate, as noise’s non-identical twin (bias) has an attractive quality, namely ‘explanatory charisma’ – when reflecting on obviously bad decisions, it’s easy to find and blame bias for all that went wrong before.
The difference between noise and bias is perhaps best explained by this diagram:
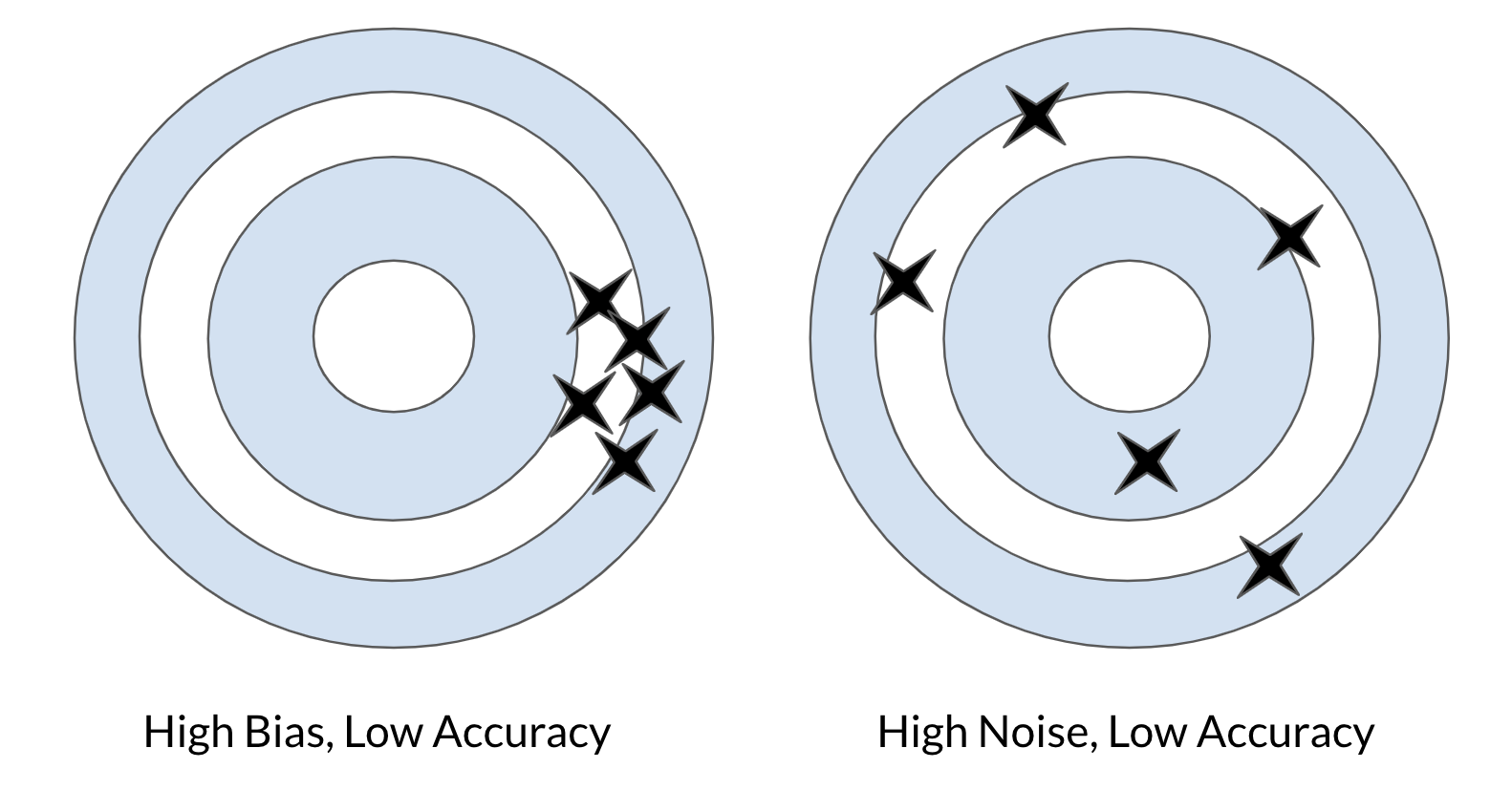
The ‘scattershot’ nature of the target on the left indicates noise, whereas the grouping on the target on the right indicates bias. Both are full of error.
Bad Times at Shackleton & Scott
Returning to the example of FinTech start-up, you’ve been tasked with getting to the bottom of the costly variability in forecasts between development teams. Being in possession of all historic data relating to estimates and delivery, you can compare forecasts with reality, thereby measuring accuracy.
You quickly discover two patterns, exemplified by the Delivery Leads Anastasia and Bharat. Team Anastasia always forecasts aggressively – on average, they estimate their work will be completed 15% quicker than actual performance. This is evidence of bias. By contrast, Team Bharat is all over the place – sometimes overestimating sales and sometimes underestimating. This is evidence of noise.
What should you recommend to Team Anastasia? Kahneman et al say that leadership should use Ex Post (or Corrective) Debiasing – essentially, to account for their planning fallacy, they should add a 15% contingency to all her forecasts.
For Team Bharat, work is required to better understand the process (or lack thereof) by which they’re calculating their estimates. For example, on what data sources are they relying, and how are they treating that data?
Leadership may be tempted to think that the variations in Team Bharat’s forecasts will ‘even themselves out over time’. That would be to misapprehend the impact of error in a noisy system. For one, the consequences are typically asymmetrical – consider the difference between being five minutes early for a train as opposed to five minutes late. At Shackleton & Scott, delivering major capabilities early is a happy problem to have – missing deadlines and releasing buggy and/or disappointing new versions of the software is not. In fact, it’s enough to persuade financial backers to withdraw their support from the business.
There are Different Types of Noise
One explanation of why the ‘noisiness’ of Team Bharat’s decision-making has gone undiagnosed is that we tend to assume that others see the world exactly as we do. That is, it might never have occurred to Team Bharat that the way they create their estimates is entirely different to their colleagues’ approach. However, it seems fair to say that if you gave the same information about the same feature to three different teams, they’d most likely generate three completely different estimates. This is the epitome of System Noise – the undesirable variability in judgments made by different people on the same issue.
But that’s not all. Occasion Noise is also present during the moment the judgment is made. This type of noise affects all our judgments, all the time. Put simply, you’re not always the same person, and you’re less consistent than you think you are. Occasion Noise can be triggered by the time of day (I get tired during the afternoons), the weather outside (I’m irritable when I’m hot), or any of a hundred other factors… Essentially, Occasion Noise acknowledges that the same individual, given the same information, on different days and under different circumstances will make different judgments. Humans are inherently noisy creatures.
Given all this, Kahneman et al argue that once noise in a system has been found, the best next step is to assess the quality of the decision-making process. They call this ‘Decision Hygiene’.
Lesson Two: ‘The Wisdom of Crowds’ and ‘The Crowd Within’
One of the simplest and most effective ways to improve Decision Hygiene is to gather other opinions – in other words, to prevail on the wisdom of the crowd. The most famous example of this phenomenon in action occurred during a country fair in 1906, when 800 people tried to guess the weight of an ox. Astonishingly, the median guess was within 1% of the actual weight. The authors of Noise are careful to remind the reader that each participants’ guess was kept hidden (or independent) from the other members of the crowd. This is because group decision-making is susceptible to being influenced by such factors as who speaks first, who speaks last, who speaks confidently, who’s sitting next to whom… Indeed, the authors point out that:
“…crowds follow tyrants, fuel market bubbles, believe in magic and can be held under the sway of a shared illusion… Chance variation in a small number of early movers can have major effects in tipping the judgment of large populations.”
And there’s more. Consider this simple question: to what extent do you agree with yourself?
To explore this amusing idea, the authors use a prompt: can you guess how many of the world’s airports are in the US? (The correct answer is included at the end of this article). Write down your first answer and don’t show it to anyone. Now assume that your first estimate was wide of the mark. Why might that be? What might you have missed? What might these new considerations imply? Was your first estimate too high or too low? Based on all these considerations, make a second, alternative estimate – and make it as different as you can.
Across a range of rigorous experiments, it’s been proven that an average of the two guesses is statistically closer to the correct answer than the first on its own. This is called ‘The Crowd Within’, and its potential ramifications for decision-making are considerable. How much better would our judgments be if we simply made a second guess?
Another chilling thought. Many of us, when tasked with making a major decision, are asked to participate in long workshops involving multiple stakeholders representing a broad spectrum of functions. The explicit aim is to gather perspectives, discuss options, and then reach a consensus (typically by voting) on the way forward. What if – despite our best intentions – these workshops are nothing but factories of Groupthink? What if early on someone poses a facile and convenient solution to the problem, around which the rest of the discussion coalesces? How much better would the outcomes be if each participant was interviewed separately, using the same questions, and the spontaneous points of agreement were used to inform the next steps?
However counterintuitive this sounds, independence is a prerequisite for the wisdom within and of crowds – otherwise “social influences reduce group diversity without diminishing the collective error”.
Scapegoating Noise as Bias
Whilst reading Noise, it occurred to me that if you ever tried to introduce these ideas to an organisation, certain objections would emerge. The most unhelpful would be the denial of bias (which is painful to admit but relatively straightforward to mitigate) and labelling it instead as noise (which can be just as important as bias when measuring the process and outcomes of judgment, but is far more elusive and difficult to tackle).
To return to Shackleton & Scott, let’s imagine that Cassandra, the HR Director, has spotted a recent spike in resignations amongst a certain cohort: female employees in their mid-to-late thirties. When she raises this during the next Exec meeting, her fellow Board members are quick to dismiss her finding, saying that ‘people leave all the time’. In this way they’re explaining away the issue as noise – resignations are common and random and often down to particular and peculiar circumstances. But their argument might actually be a tactic to hide the level and type of bias being exposed. At Shackleton & Scott, the gender split below the Senior Developer level is 80% women, 20% men. At Senior Developer level and above, the split inverts: 80% men, 20% women. Female professionals in their mid-30s are leaving because of a lack of career opportunities, due to the fact that male hiring managers are hiring male candidates – a clear and obvious example of bias.
Lesson Three: Humans Are Not Better Than Machines
Another way of significantly improving Decision Hygiene is to remove, or at least reduce, noise by using ‘simple, mechanical rules’. Across a set of ambitious experiments, American Psychologist Paul Meehl proved a highly controversial point: algorithms are generally superior to human judgment.
Before exploring that controversy, we should pause for a moment to consider the word ‘simple’. The authors of Noise use the example of trying to calculate the probability of someone skipping out on bail. In the set of ‘simple, mechanical rules’ guiding the decision-making, the first variable was age (people who jump bail tend to be younger), and the second was the number of past court dates missed (or, the rate of recidivism). When tested, this two-factor model matched the validity of another tool which incorporated 137 variables. The lesson is that selecting the correct variables to inform your decision-making process is vital – and that more isn’t necessarily more.
Returning to Meehl’s finding that algorithms are better than humans at making decisions, the idea is disputed for a number of reasons. One is that bias is built into algorithms. Certainly, if past source data replicates human prejudice, an algorithm can aggravate and/or perpetuate this prejudice (although the authors are quick to point out that it can also be instructed not to). But it stands to reason that algorithms are not automatically unfair, biased and/or discriminatory.
Beyond this point, the question of why algorithms have such a bad reputation isn’t explored in any depth in Noise – the authors are uninterested in this debate, and/or consider it beyond the scope of their argument. However, since reading the book it’s occurred to me that another reason the value of decision-making algorithms is denied is because they endanger the jobs of knowledge workers. Thinkers such as the late David Graeber are quick to point out that those who own the factors of production love to automate ‘menial’ tasks and find efficiencies by firing unskilled workers. But when it comes to the safety of their own jobs, it’s a different story entirely…
Ghosts in the Machine
One of the most interesting case studies in Noise concerns the introduction of Machine Learning to the recruitment process at a large tech company. After the algorithm was trained on over 300,000 submissions previously received and evaluated, it was then tasked with screening the CVs of software developers to identify the best candidates. Compared to its human counterparts, the algorithm selected candidates that were 14% more likely to receive a job offer, and 18% more likely to accept said offer. Also, the Machine Learning-derived shortlist was more diverse in terms of race, gender, educational background and employment history. And yet despite these encouraging results, as far as I know, no companies have announced that they’re replacing Tech recruiters with Machine Learning. Why?
The answer appears to be that people don’t trust machines to make these kinds of decisions. The latitude afforded to error-prone humans is not extended to machines. This can be attributed to two main factors:
- The level of accuracy between humans and machines is considered too narrow. According to the research, the difference in aggregate settles at around +5%
- The machines are not perfect (yet)
So, whilst algorithmic models deliver consistently better results than people, they’re not that much better. And at first glance, 5% doesn’t sound like much, but imagine 5% more conversions through an organisations’ sales funnel. Such a gain would revolutionise most businesses that routinely invest in far more incremental and/or uncertain improvements. And the difficult truth is that perfect prediction is impossible – the present, let alone the future, is far too complex and uncertain. The authors wryly note: “the obviousness of this fact is matched only by the regularity with which it is ignored.”
Lesson Four: Why ‘Gut feel’ Persists in the World of Decision-making
It’s hard to admit you’re wrong – or worse still, to admit that you don’t even know if you’re right or wrong. Acknowledging this feeling of ignorance is painful, whereas acting on instinct delivers an instant and powerful emotional reward. It’s no wonder that ‘gut feel’ persists.
As humans, we like all the pieces of the puzzle to fit together neatly. We see patterns everywhere, hence our ability to see faces in clouds or in burnt toast. Typically we achieve coherence by ignoring any evidence that doesn’t quite fit with our desired narrative. Decisive leaders erase uncomfortable and inconvenient truths, thereby inspiring certainty amongst others. Indeed, intuitive decision-making emerges most prominently when the situation is at its most uncertain. When solid facts are absent, intuition rushes the vacuum, providing the confidence people crave.
Exacerbating this phenomenon is the overpoweringly persuasive and seductive concept of ‘following your instincts’. Over and over again we’re told stories about singular genius being undone by compromise and consensus, whilst individuals brave enough to resist the crushing weight of the status quo are able to make giant leaps forward. The archetype of unreasonable genius pursuing progress, for its own sake and at any cost, is Dr Victor Frankenstein – but in more recent times we also have Elon Musk and Steve Jobs, who through the force of their personality make the world turn around them.
But the power of gut feel is a myth. It relies on the decision-maker being able to remake the past into a coherent, causal system in which all their mistakes are forgotten and all successes are of their own making. This contributes to overconfident predictions about the future, and without compensating systems and processes, can pave the road to disaster.
Lesson Five: Why it’s so Hard to Reduce Noise
As we’ve discussed, using intuition is fun and intrinsically rewarding, whereas following a more mechanical process is not (it reeks of bureaucracy, red tape and delay). The Economist Albert Hirschman identified three principal objections to reforming a system:
1. Perversity – the solution only aggravates the problem
2. Futility – nothing changes
3. Jeopardy – interventions unintentionally endanger other important values.
At Shackleton & Scott, you recommend that Team Bharat follow a new, rigorous process by which they calculate their estimates. They feel offended by the insinuation that they’ve not been doing a very good job, and as a consequence become resentful and careless in their work. This would eventually lead to even more noise in their judgments, and more inaccurate estimates (an example of perversity). Alternatively, Team Bharat commit to following the process to the letter, despite finding it tedious in the extreme, and their estimates still remain unpredictably variable (an example of futility). Finally, Team Bharat try their best to reduce the noise in their estimates but in the attempt, lose too much autonomy, which leads them to quit the company to join a rival, taking all their domain knowledge and expertise with them (an example of jeopardy).
As ever, there are trade-offs to consider when it comes to improving Decision Hygiene. Hard and fast rules constrain judgment and reduce noise, but can provoke endless arguments (for example, “We should take down all public statues of historical figures”). Producing defensible rules that apply consistently in the long run and to all cases is hard, if not impossible. They also establish a fixed position that can be endlessly challenged by edge cases (for example, what about the statue of Emmeline Pankhurst in Manchester?). On the other hand, open-ended guidelines remain open to interpretation and are therefore noisy (for example, “We should only take down public statues of historical figures we deem offensive”). Exercising judgment on which cases are on which side of the line is burdensome, slow and wearying for those called upon to make those margin calls. Those decisions are also susceptible to the old enemy bias.
Conclusions
Here are the three main lessons I’ve learned from reading Noise.
When making decisions I should:
- Collect a diverse set of independent perspectives – sharing perspectives too early runs the risk of Groupthink. Group discussions can also be influenced by early, charismatic contributions
- Resist being seduced by gut instinct in the presence of uncertainty, and instead employ simple mechanical rules built around a small number of carefully selected variables to anchor my thinking
- Focus on the quality of my Decision Hygiene – what biases are warping my judgments, and how might they be corrected? What rules should be applied, and when are guidelines more suitable?
I hope this article was useful, and good luck in your efforts to reduce the amount of noise in your judgments!
**The US has 32% of the world’s airports. Were you closer to the correct answer with your first or second guess?**
***
If you or your CTO / technology lead would benefit from any of the services offered by the CTO Craft community, use the Contact Us button at the top or email us here and we’ll be in touch!
Subscribe to Tech Manager Weekly for a free weekly dose of tech culture, hiring, development, process and more